DeepSeek R1: A Hedge Fund's Side Project Disrupting the AI Landscape
A Sputnik Moment in AI Development
DeepSeek, a Chinese AI startup founded in 2023, has burst onto the scene with the unveiling of its R1 model on January 20, 2025—a development that some are calling AI’s “Sputnik moment.” The company’s dramatic entrance began when it claimed its new system can match or exceed the performance of established heavyweights like OpenAI’s ChatGPT and Google’s Gemini, yet at a fraction of the hardware and cost requirements—purportedly using only about 2,000 Nvidia chips instead of the 16,000 typically employed by larger U.S. tech firms. Adding fuel to the buzz is DeepSeek’s decision to fully open-source R1, including its underlying weights, a move that has sparked both excitement over democratizing AI and skepticism about the model’s true development costs and the true intent of the company.
Immediate Financial Ripples
Financial markets wasted no time responding to DeepSeek’s announcement. By January 27, the Nasdaq index had dipped around 3.1–3.4%, while Nvidia’s shares tumbled between 12% and 17%, erasing approximately $590 billion in market capitalization. Micron Technology, ASML, and others also saw losses of 7% to 9%, culminating in the S&P 500 tech sector’s most severe single-day decline since 2022. Although it is reasonable to question DeepSeek’s ability to make inroads into U.S. markets, the company’s overnight disruption of the AI scene has unsettled investor confidence and reignited debates over whether American companies are overspending on infrastructure.
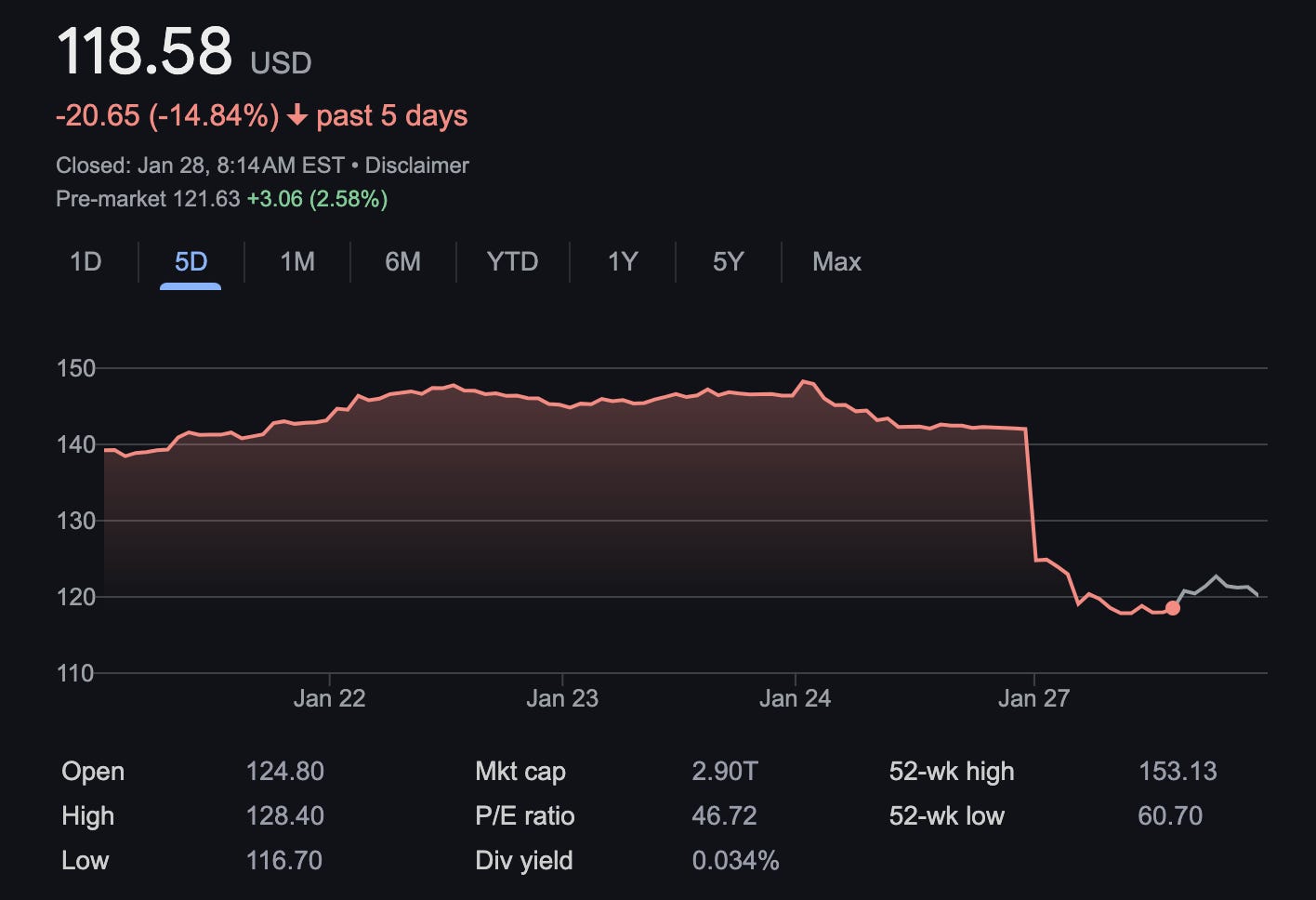
Excitement over R1 has been swift among end users: DeepSeek’s app rocketed to the top of Apple’s App Store within days. Meanwhile, the company reported large-scale cyberattacks, prompting it to temporarily cap new user registrations, making some wonder if the attacks reflect tensions around releasing a powerful AI tool at no cost—particularly one rooted in Chinese research. Regardless, DeepSeek’s rapid rise has sent the AI community scrambling to reassess assumptions about cutting-edge performance, high-budget infrastructure, and what it will take to stay competitive in a quickly shifting global landscape.
DeepSeek R1: Side Project of a Hedge Fund
DeepSeek R1 was developed as a so-called “side project,” an initiative propelled by DeepSeek’s origins as a quant hedge fund called Fire-Flyer. Founder and Zhejiang University alumnus Liang Wenfeng had reportedly stockpiled substantial quantities of Nvidia A100 GPUs prior to U.S. export restrictions. Despite operating under comparatively modest resources by Western standards, DeepSeek claims to have trained R1 for only about $6 million—a stark contrast to the hundreds of millions of dollars reportedly spent by companies such as OpenAI on comparable models.
This startling cost efficiency has sparked intense debate about how an AI model capable of near human-like reasoning, coding, and mathematics could be developed at such a fraction of the expense. Some see DeepSeek’s willingness to give away a sophisticated system as a strategic move that could disrupt U.S. tech powerhouses’ business models. Others question the transparency of DeepSeek’s methods and worry about implications for American AI competitiveness. It is also significant that R1’s release came on the heels of substantial U.S. investments in AI infrastructure—a $500 billion initiative called Stargate announced by President Trump last week, designed to bolster next-generation systems.
People are divided about the motives behind releasing a powerful model for free. Skeptics label it a Chinese ploy—rumoring that state-backed funding or government incentives might be involved—aimed at undermining American AI leadership. More optimistic voices see it as a leap toward democratizing AI research, echoing venture capitalist Marc Andreasson’s position that open access can significantly benefit humanity. These opposing perspectives underscore how unpredictably AI breakthroughs can alter the global tech balance and elevate international competition.
DeepSeek R1’s Capabilities and Cost
From a technical standpoint, R1 introduces what DeepSeek terms “test-time compute,” suggesting the model can optimize its thinking as it responds to queries. Many have been astonished that R1’s performance seems comparable to, or slightly better than, OpenAI’s 01 model, which reportedly cost hundreds of millions of dollars to train. DeepSeek’s background as a quant hedge fund explains how its GPU resources might have gone unnoticed. The company could have repurposed hardware originally intended for high-frequency trading strategies. This move likely reduced the overhead of building a large-scale model from scratch. Naturally, many wonder how DeepSeek might monetize its accomplishment after handing out the model for free. The revelation that a “side project” could rival the largest Western AI labs has rattled conventional wisdom about resource requirements and capital allocation in AI.
Report are already emerging that DeepSeek’s AI assistant avoids topics considered sensitive in China, reinforcing existing tensions between technological openness and political oversight in the country. DeepSeek’s claim of achieving top-tier results with only a fraction of the hardware further raises eyebrows: does it receive undisclosed state support or have hidden resource pools? The company’s finances also remain opaque, fueling doubts while many are asking if American firms’ multi-billion-dollar outlays on specialized AI clusters are truly necessary. Emad Mostaque, founder of Stability AI, has examined DeepSeek’s hardware usage and found the numbers plausible; indeed, he theorizes that costs could drop below $2.5 million if more advanced H100 GPUs were used.
Leading American companies like OpenAI, Meta, and Microsoft, which have invested billions in AI, now face mounting pressure to justify their enormous spending. Adding to the scrutiny is DeepSeek’s remarkable claim of processing over 200,000 API requests for just 50 cents—fueling speculation about the efficiency and scalability of its model. If operating at such low cost, DeepSeek underscores the transformative potential of test-time compute as a future inflection point in AI. However, questions remain about whether R1, DeepSeek’s model, truly relies on minimal hardware or if a “hidden armada” of GPUs supports its operations behind the scenes. This uncertainty has sparked debates among investors like Chamath Palihapitiya, who points to Jevons’ Paradox—the idea that greater efficiency often drives higher consumption. Should other AI firms adopt DeepSeek’s resource-efficient approach, global AI usage could surge, intensifying competition for compute resources and bolstering long-term demand for companies like NVIDIA.
In a field that values maximum performance, consistent access to cutting-edge compute, data, and hardware is likely to remain a decisive factor in determining industry leaders. Palihapitiya also raises concerns about the geopolitical implications of R1, particularly if China has circumvented export controls to secure advanced hardware. He contends that the U.S. is entering the era of inference, where large-scale practical AI products can proliferate rapidly if inference chips are widely accessible. To maintain its leadership, he argues, America must attract global customers to U.S.-origin AI solutions, winning the trust and loyalty of users worldwide.
A Important Moment in AI
The release of R1 underscores the volatility of the AI landscape, where each breakthrough has the potential to upend established norms. By open-sourcing R1, DeepSeek has made its system accessible worldwide, offering capabilities that rival those of well-funded U.S. labs. This bold move raises critical questions about competitiveness and the regulation of advanced technologies across borders.
On the surface, R1 challenges long-held assumptions that only heavily financed Western corporations can produce cutting-edge AI. That a Chinese firm succeeded with older GPUs and minimal resources disrupts traditional notions of resource dependence and dominance. The market’s response underscores how much current valuations hinge on AI’s unrelenting progress.
For the United States, the key challenge lies in adaptability. Policies must prioritize advancing next-generation hardware, nurturing AI talent, optimizing the use of relevant data assets, and implementing export controls that balance security with global collaboration.
While skepticism about DeepSeek’s claims is warranted, R1’s release has undeniably widened the competitive landscape. Whether it signals a new era of Chinese AI capabilities or the power of open-source innovation, it serves as a wake-up call—a reminder that the next wave of technological progress may emerge from unexpected places, prompting us to ponder who may control the future of AI and urging us to remain vigilant.