For over a century, cancer classification has relied heavily on identifying the organ of origin and examining histological features under a microscope. In a recent perspective published in Clinical and Translational Science, I argued that while this traditional approach has been foundational, it is increasingly misaligned with the needs of modern precision oncology. I proposed that artificial intelligence (AI), particularly machine learning and deep learning techniques, could bridge this gap by integrating multiple data modalities to create more biologically meaningful classification systems.
This shift is exemplified by recent work from the Human Tumor Atlas Network, published in Nature, which demonstrates the kind of sophisticated analysis needed for next-generation cancer classification. Their study analyzed 131 tumor sections across six cancer types, integrating high-resolution data from Visium spatial transcriptomics, single-nucleus RNA sequencing, and CODEX multiplexed imaging. This analysis brings us closer to a more nuanced understanding of cancer’s complexity.
As I discussed in my perspective, tumors originating in different organs can share critical molecular and morphological characteristics, while tumors within the same organ can exhibit significant heterogeneity. The Atlas Network’s findings reveal this complexity at a granular level, identifying distinct “microregions” within tumors—spatially distinct clusters of cancer cells separated by stromal elements. These microregions possess unique genetic clones, each exhibiting specific copy number variations and varying levels of oncogenic activity—precisely the type of biological complexity that traditional classification methods often overlook.
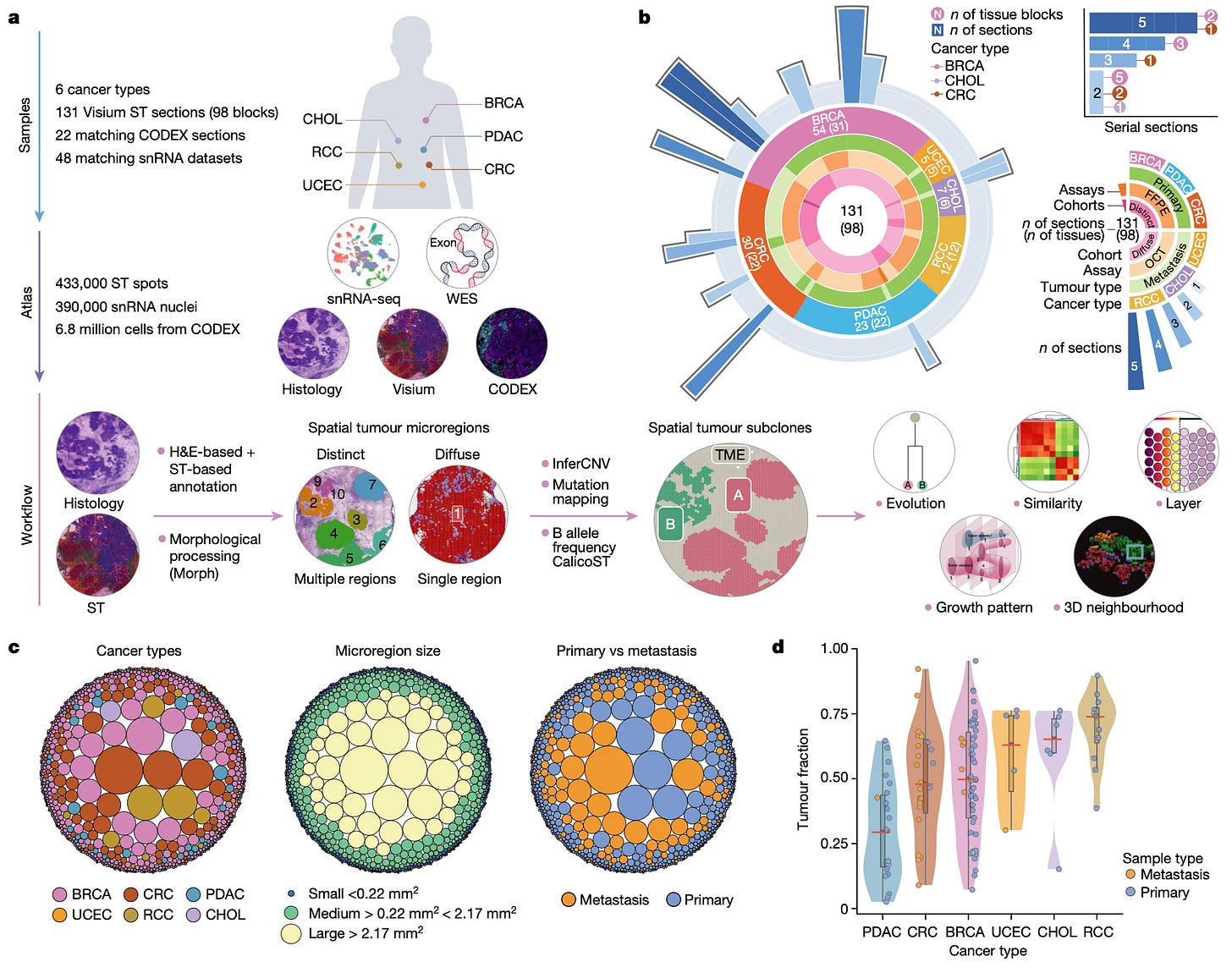
The Atlas Network’s implementation of advanced computational methods represents an essential step toward an integrated approach to understanding malignancies. Through spatial alignment and analysis of 48 serial tissue sections, their three-dimensional reconstruction of tumor structures revealed spatial architectures with submicron resolution. This enabled them to quantify microregion density, connectivity, and cellular composition across the tumor volume. Such high-dimensional spatial analysis generates the complex data matrices that machine learning techniques can analyze, identifying patterns beyond human visual and cognitive capabilities.
Their findings on treatment resistance further underscore why we need to move beyond conventional classification systems. They observed that treatment-resistant subclones often form larger, metabolically active microregions, with increased immune exhaustion markers at their peripheries. The presence of both immune-hot and immune-cold neighborhoods highlights biological features that can inform quantitatively defined modern cancer classification efforts.
Advancing precision oncology requires embracing the full complexity of tumor biology through AI-enabled multimodal analyses. By correlating morphological features with genomic, transcriptomic, and proteomic data, AI models can facilitate more nuanced classifications that better predict patient outcomes and therapeutic responses. Integrating spatial organization, genetic heterogeneity, and microenvironment interactions is essential to deepen our understanding of cancer. This evolution in cancer classification has can significantly enhance treatment selection and improve patient outcomes, bringing us closer to the goals of precision oncology.
The progress made by groups like the Human Tumor Atlas Network paves the way for this future. Their detailed 3D reconstructions of tumor volumes reveal connectivity and microregional interactions, illustrating how a deeper understanding of tumor architecture can inform more precise therapeutic interventions. As we continue to gain insights into tumor complexity and develop increasingly sophisticated analytical tools, we move closer to classification systems capable of effectively guiding precision medicine. The convergence of spatial biology, molecular profiling, and AI presents unprecedented opportunities to transform our understanding and treatment of cancer. The future of cancer classification lies not in static anatomical categories but in dynamic, AI-driven analyses that capture the true complexity of oncogenesis, disease spread, maintenance, and resistance mechanisms.
Seems like cost (and complexity) will be rate limiting for clinical adoption since deep measurement and deep analysis now possible.