The Science We Thought We Knew
Redefining Precision in Biomedicine with AI-Driven Probabilistic Models
Science has never been a fixed body of knowledge, nor has it been governed by immutable gold standards. It is a process of continual refinement—an evolving series of approximations, always subject to revision in the face of new evidence. Medicine has long relied on structured frameworks that reflect this principle, even if they were mistakenly perceived as definitive. Cancer staging, for example, has shaped clinical decision-making for decades, offering a practical, albeit simplistic, means of classifying disease. But staging has always been more artifact than absolute, an imperfect system shaped by human expertise and constrained by the tools available at the time.
So where do we stand now, as artificial intelligence infiltrates nearly every aspect of public discourse? What is the thread of truth amidst the noise? AI is neither a cure-all nor a disruption for disruption’s sake. Its power lies in its ability to challenge our assumptions—not by declaring new absolutes, but by revealing that the frameworks we’ve long considered gold standards were never truly standards at all. They were approximations, and they can be better approximated. In medicine, this means moving beyond static classifications toward inference-driven models that evolve as our understanding deepens.
The Fallacy of Fixed Standards
For decades, clinical oncology has relied on classification systems built on anatomical and histopathological observations. These methods, though practical in their time, function as broad filters, reducing complex biological systems to categories that were never meant to be definitive. Two tumors classified as “lung cancer” may be genetically and functionally distinct in ways that profoundly affect prognosis and treatment response—differences that traditional staging and diagnostic methods cannot capture.
Beyond their biological limitations, these systems are riddled with human variability. Studies show that radiologists assessing the same tumor scan disagree at least 30% of the time, and oncologists must often rely on subjective interpretations to bridge the gaps left by rigid frameworks. Even RECIST, the so-called gold standard for measuring tumor response, operates on unidimensional size-based criteria that fail to reflect spatial, functional, and molecular evolution. The persistence of these tools is not evidence of their infallibility—it is simply inertia, a reliance on what has worked well enough despite its known flaws.
But medicine cannot afford to be built on “well enough.” The idea that there are true gold standards in biomedicine is an illusion—science does not deal in absolutes, only in models that approximate reality with varying degrees of precision. And when those approximations improve, we must be willing to evolve with them.
AI as an Engine of Refinement
AI does not introduce certainty—it introduces better probabilities. For example, unlike static classification systems, AI-driven models can integrate vast amounts of multimodal data—genomics, imaging, clinical records—to generate inference-based assessments of tumor behavior. They do not offer fixed conclusions, but rather continuously refined probabilities that shift as new information becomes available.
Our PRECISE initiative at Project Data Sphere exemplifies this shift. By employing autonomous tumor detection, volumetric segmentation, and molecular profiling, we are working on a novel Total Tumor Burden Index (TTBI)—a metric that evolves in real time as a tumor progresses or responds to treatment. TTBI is a fundamental reframing of what precision means: not a static label, but a dynamic estimation of disease burden. AI-driven models like TTBI don’t claim to be gold standards—they acknowledge that there is no such thing. Instead, they offer increasingly accurate approximations, shifting with the complexity of biological systems rather than forcing them into pre-defined categories.
This is not a rejection of traditional oncology and medicine; it is an expansion of it. AI may not yet be a superior alternative to human expertise, but it is a reflection of science’s true nature—a recognition that we do not deal in certainties, only in progressively refined inferences.
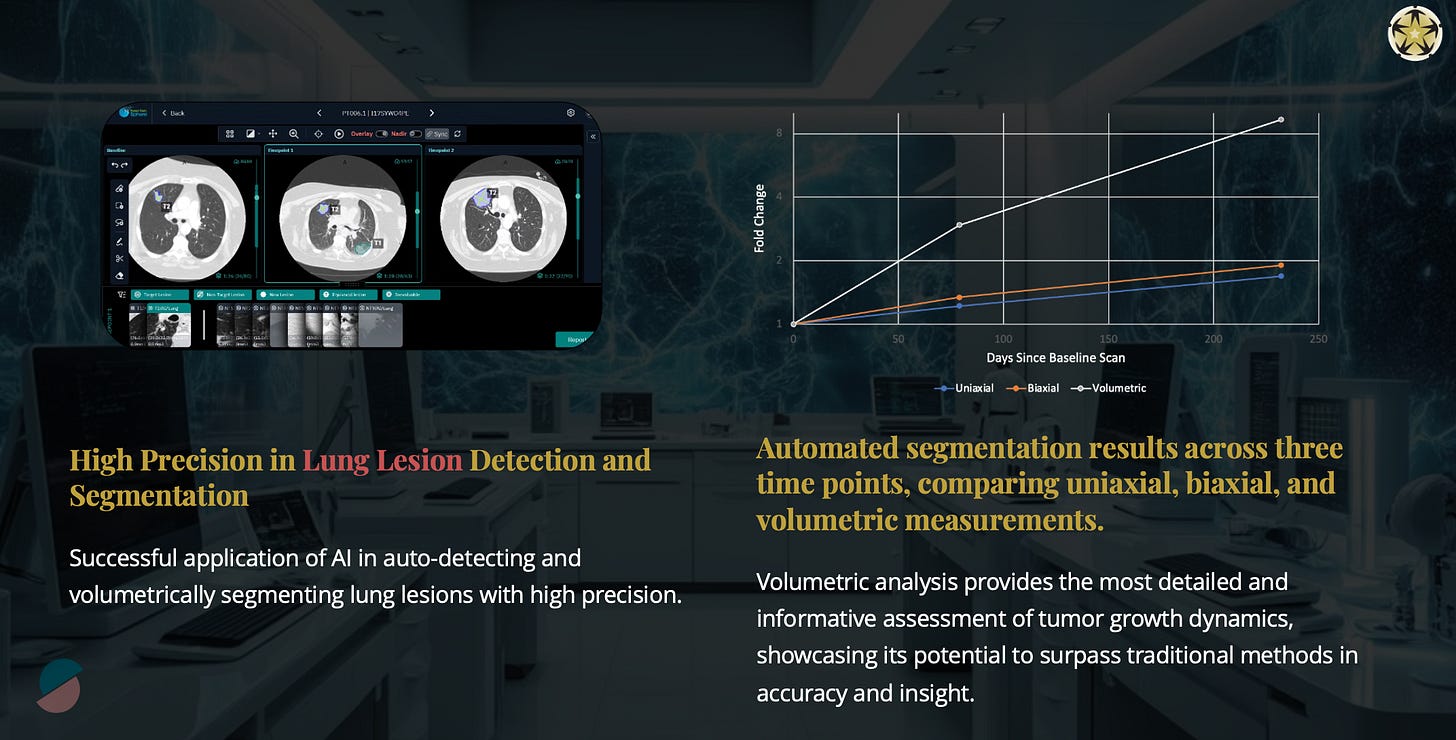
Rethinking Precision as a Moving Target
We must not think of integration of AI into biomedicine as just a technological shift; but more of a conceptual shift. It forces us to reconsider the idea that precision medicine has an endpoint or that any framework can serve as a permanent guidepost. Traditional systems, while still valuable, must be seen for what they are: functional approximations that were never meant to be static truths.
This is also critical in clinical trials, where rigid cohort definitions based on outdated staging systems lead to inefficiencies and suboptimal patient selection. AI-driven models offer a different approach—not by imposing new universal rules, but by allowing patient classification and treatment decisions to be continuously refined based on real-time biological insights. The result is not just better science, but a better philosophy of science—one that acknowledges uncertainty and adapts to it, rather than resisting it.
Medicine has long relied on categorical truths because they provided structure, but structure is not the same as accuracy. AI allows us to move beyond the illusion of fixed classifications, making precision medicine an ongoing process rather than a one-time determination.
The Future Demands Adaptation
The greatest fallacy in science is the belief that its frameworks represent finality. There has never been the science, only the pursuit of better approximations. In medicine, this means recognizing that, for example, traditional classification systems, while useful, were never gold standards—they were stopgaps, shaped by the limitations of their time. AI offers a path forward not because it delivers certainty, but because it embraces uncertainty, refining our understanding through dynamic, inference-driven models that continuously evolve.
To resist or fear this transformation is to misunderstand the very nature of science. Precision medicine must not be a fixed endpoint but an adaptive process—one that continuously integrates new insights to refine diagnosis, treatment, and research. The integration of AI into biomedicine does not render traditional methods obsolete; it forces us to see them for what they always were: approximate, functional, but ultimately incomplete. The time to adapt is now—not because AI is inevitable, but because evolution in science has always been.
There are no gold standards, only better questions waiting to be asked.