Unearthing Mechanistic Foundations of Oncological Diseases with AI
From artifacts of history to precision medicine
Precision medicine has brought about a new era in the treatment of cancer with the goal of giving the right drug to the right patient at the right time and in the right dose and intensity. However, as we delve deeper into the intricacies of cancer treatment, it becomes increasingly clear that standard diagnostic criteria can fall short in identifying the right patient. The traditional approach, which places anatomy at the top of the hierarchy of disease classification, is largely an artifact of history based on subjective definitions derived from human sensory input and articulated in rather arbitrary linguistic terms. For example, visual inspection of a biopsy specimen tethered still on a glass slide bathed in paraffin and dye with standard microscopy for classification of non-small cell lung cancer produces very little information about the underlying mechanisms of disease. The term "non-small" itself has more of a literary flair than a scientific one and I'm increasingly considering such terms as surrogates attempting to predict much deeper phenomena with varying degrees of precision that typically manifest themselves as heterogeneity in patient outcomes. Striving for more precise characterization of disease based on clearly defined parameters such as shared underlying pathophysiology can enable classifying patients into homogenous cohorts for the development of new therapies with highly predictable safety and efficacy profiles.
This is not to say that the existing histopathological classification schemas are not important. In fact, they're remarkable since, despite their reductionist simplicity, they contain a lot of information in defining broad phenotypes, which in some cases we've successfully rendered more precise with molecular profiling and other biomarker-driven strategies. However, the standard criteria today are also inherently subjective. At the recent Precision Medicine World Conference (PMWC) in silicon valley, I discussed the utility of AI-based histopathological image analysis for the development of objectively-derived new disease categories and therapeutic targets. More specifically, I focused on the use of AI-based imaging in identifying deep mechanistic realities that lurk under the shadows of current standards and workflows to provide a more objective diagnostic framework closer to underlying disease mechanisms, agnostic to anatomy and immune to the limitations posed by human visual interpretations of cellular morphology.Â
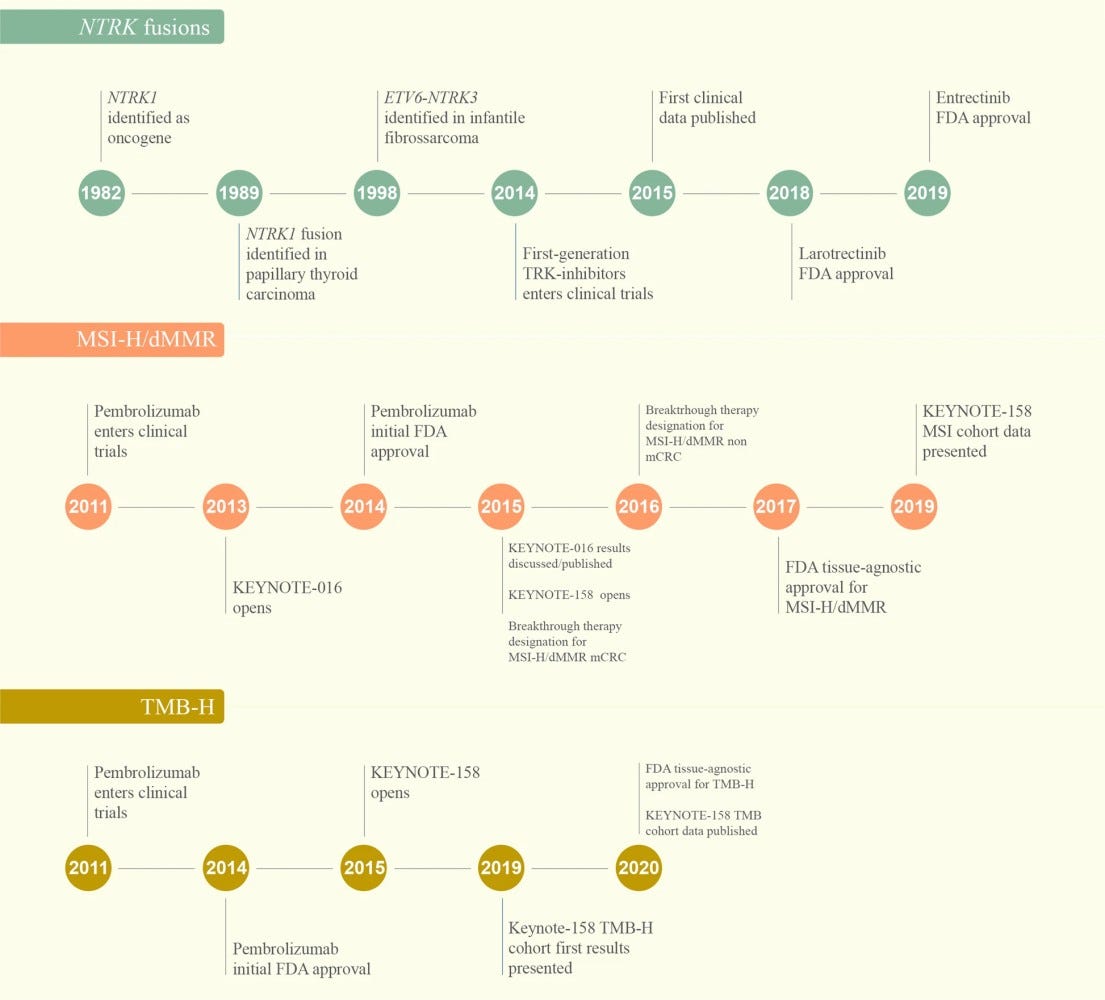
The emergence of tissue-agnostic indications can be thought of as the start of a shift in perspective towards mechanistic classification of cancers, as exemplified by the US approval of pembrolizumab in patients with MSI-H tumors in 2017. This approval occurred during my tenure at the FDA at a time when transitioning away from conventional methodologies and decreasing the significance of anatomy in molecular and biomarker strategies were at best ambiguous concepts for many stakeholders. Today, the increasing adoption of these approaches coupled with the established regulatory precedent can be foundational in facilitating AI-based mechanistic disease classification frameworks. During my presentation at PMWC, I encouraged the AI biomedical community to build upon this foundation and place more emphasis on extracting fundamental mechanistic features that can define completely new disease phenotypes. I highlighted harnessing the power of the latent space in trained AI algorithms as one promising strategy. The latent space is the mathematical representation of how AI algorithms compress and sort the data they're trained on into organized clusters that humans, even when armed with conventional analytical tools, are not able to do. Supervised or unsupervised, training of AI algorithms with appropriate datasets leaves behind a rich mathematical representation of insights to be explored. However, the majority of AI-based imaging efforts today attempt to correlate these representations to existing "gold standard" criteria that can reinforce the same logical fallacies from which traditional disease classification standards suffer.
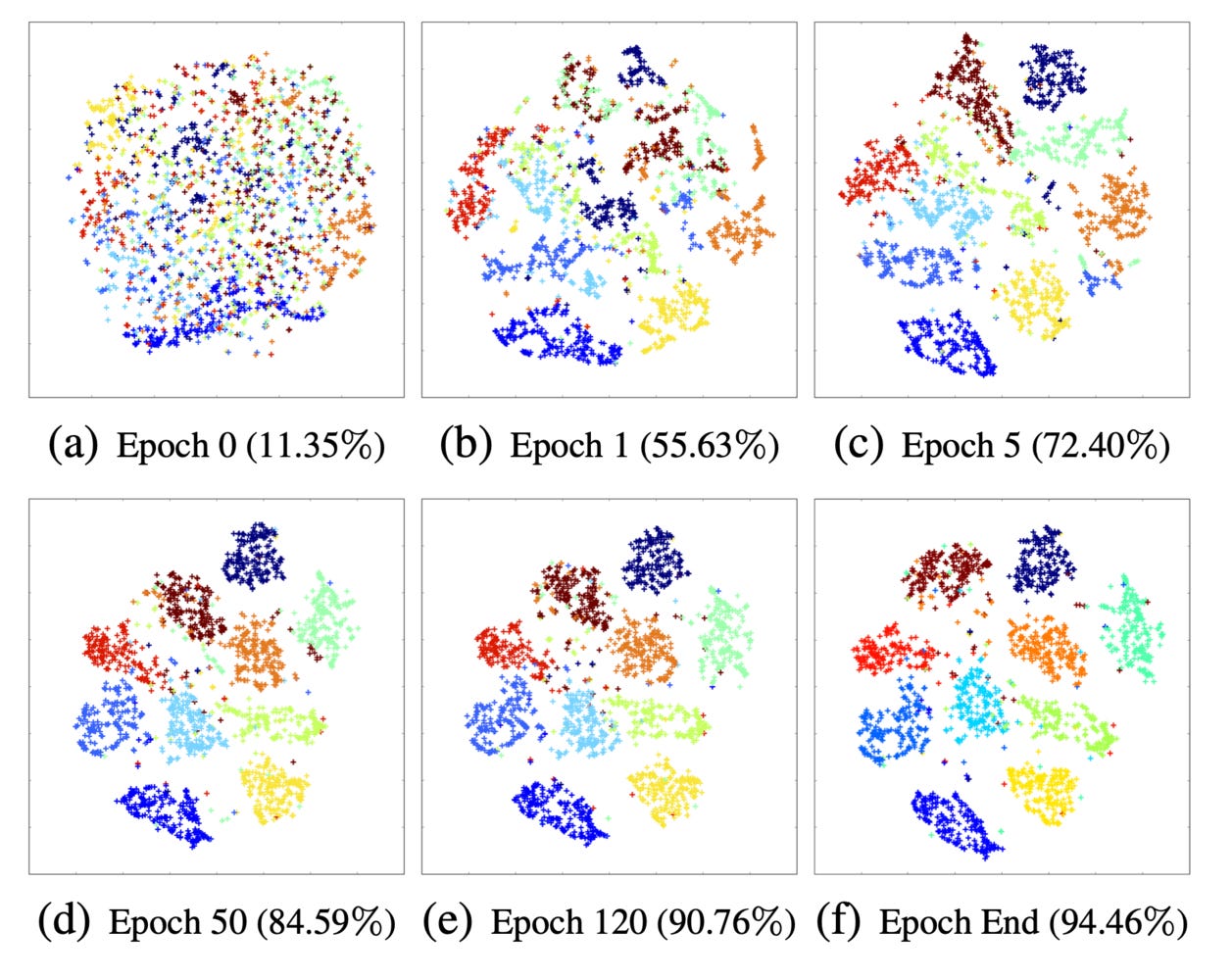
I'll continue to advocate for this approach over the next few months because I strongly believe it's a low hanging fruit for unlocking mechanistic insights for new therapeutic targets. My next stop will be the Leap conference in Riyadh next week where I'm looking forward to presenting on AI-powered computational imaging.
We live in amazing times.